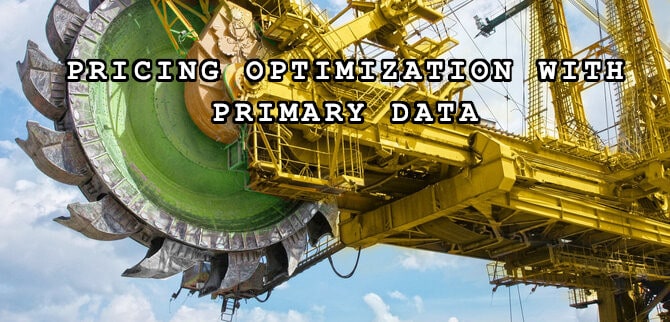
07 Oct Pricing Optimization For Physical Products Using Primary Data
Pricing optimization has the highest ROI of all the revenue growth value drivers I work with. Higher than growing market share, customer expansion, product expansion, market expansion and customer retention. Why? Because of the “I” part. Price optimization does not require an investment in product development or customer acquisition. All it requires is figuring out the price metric within a business model. Just a number. But because it’s a number that often drops out of a consultancy’s black box, it’s a number that tests the confidence of many a management team who fear losing customers and new sales if they get it wrong (too high). This fear is justified because however that price is derived, it’s supported by a wobbly house of secondary data cards.
This fear is justified because however that price is derived, it's supported by a wobbly house of secondary data cards.
Some companies use cost-plus pricing and others use competitive-based pricing, but the gold standard is value-based pricing. Value. Since price is exchanged for value, to get the price right we need to understand how much value the product produces… which is easier said than done. Today, understanding what exactly the customer values and how much they value it is a qualitative research project at best. Surveys are deployed, focus groups are assembled and discussions are had to hear how much (extra) the customer is willing to pay and which features are most important. Management believing in new pricing requires a leap of faith because no matter how clever or complex, that derivation is perched upon a few assumptions based on secondary data. Whew. Kind of prehistoric, isn’t it?
Fast forward a couple million years and we now have digital products that can measure the value they produce. By using revenue earned as a proxy for value produced (in B2B) digital twins of physical products can measure this directly by collecting revenue-associated data with the internet of things and analyzing it with AI. This begins by understanding the KPIs in your customer’s business model, and ends by determining the proportionate role your product plays in making the customer money. Choosing which types of sensors to install is based on the KPIs we need to measure.
Let’s look at two simplified examples to make this more concrete: one in the software sector and the other in the mining sector. The KPI for graphic design software, like AI generated art, is the number of images purchased. The KPI for mining extraction machinery, like a bucket wheel excavator, is metric tons of material extracted. These KPIs sit within the customers’ business models and their corresponding data, number of images and weight, can be collected by software or sensors. To complete our pricing model, we determine the product’s attribution to value through desktop work.
In this conceptual overview I only touch on price but in the future I’ll discuss how to analytically define packaging – the other key factor in capturing the full value a product produces. And this line of thinking naturally leads us into business model design, but that’s a broad topic for another time.
The pricing optimization approach I describe here is based on pure primary data – clean and unbiased. By sensing how customers make money with our physical product, pricing becomes an analytical exercise that can stand up to the scrutiny of any skeptical CFO and quell the fear of their management team. This is the definition of value pricing.
HOW IT’S DONE
To use primary customer data to optimize pricing we deploy a monetization sim. It’s purpose is to simulate how the customer makes money with the product. Like the other sims deployed, the monetization sim consists of an AI model wrapped with software to feed it data. It is inferenced through a web interface.
Instead of a pricing calculation this is a living multivariate AI model. Meaning this is not a set and forget value/price since price is constantly being refined over time as more data is collected and the sim is improved. Nor is this a one price fits all solution as we collect data to calculate price from all customers allowing us to dynamically derive the best price for each ideal customer profile (ICP) within each customer segment.
Contact us if you’d like to understand more or to schedule a free 30-minute consultation if you may have a physical company that could fit this type of pricing optimization.