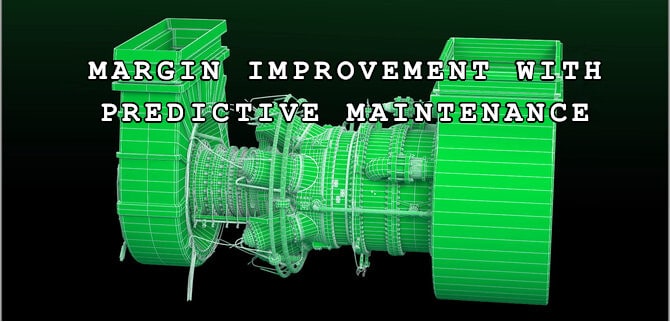
04 Apr Margin Improvement with Predictive Maintenance
Revenue growth has more impact on enterprise value than margin improvement. It’s a fact. For a midsize company with 36% gross margin and 17% profitability, a 1% increase in revenue results in a 6% increase in EBITDA. That, compared to a 1% reduction in variable costs and fixed costs results in a 3.8% and 1.1% increase in EBITDA respectively. Having said that, increasing profit margins is still a go-to private equity move because, one, it’s old-school-tried-and-true, and two, it’s more controllable than growing revenue. Why? Because reducing costs is in your control, so you know what you’re going to get. But with revenue growth you can’t entirely predict what the customer will do since they’re out of your control. It’s more like pushing a rope than pulling a lever.
Smart digital is behind two recent trends that are reducing maintenance costs. The first is moving from onsite repair to remote repair, and the second is the transition from proactive to preventative to predictive maintenance.
Margin improvement comes in many different flavors. Some are an indirect consequence of revenue growth and others are more direct in nature. Indirect value drivers, all of which I’ve discussed here before, include customer retention and customer expansion which both lower customer acquisition costs, product expansion into higher margin products, market expansion into higher margin markets and pricing optimization which when raised (it’s always raised) naturally expands margins. However, when most pros talk margin improvement, they mean direct activities such as cost cutting and increasing operational efficiency. The latter is where I’ll go here, that is, how to use smart digital to increase operational output while using the same resources.
Choosing which operational group to make more efficient depends on the company’s KPIs and strategic objectives. Generally this points to the operations that make the company money. If the company makes things, the cost of goods is lowered. If the company services things, the cost of service is lowered. If the company operates things, the cost of operations is lowered. If the company sells things, the cost of customer acquisition is lowered. And so on.
As an example, consider predictive maintenance as used in the manufacturing and industrials sectors. Predictive maintenance reduces the cost of manufacturing for which the cost of goods is a good measuring stick, but there are others. Smart digital is behind two recent trends that are reducing maintenance costs. The first is moving from onsite repair to remote repair, and the second is the transition from proactive to preventative to predictive maintenance.
Predictive maintenance uses AI to predict when a piece of equipment or a machine, or any type of asset will fail. To maximize asset utilization, a subset of operational efficiency, the goal is to only stop to fix assets during scheduled maintenance periods – anything else is a costly disruption. For example, if a manufacturing line is scheduled to stop for maintenance the first day of each month but we predict a piece of the line will go down in the middle of the month, then we schedule its repair at the beginning of the month it’s predicted to fail in. By fixing the problem in advance, during the planned downtime, we reduce unplanned downtimes – the nemesis of manufacturing margins.
In businesses where maintenance has a meaningful impact on margins, we can use artificial intelligence to predict when assets will fail in advance of any noticeable signs of a problem. By using smart digital to prevent unplanned downtimes, we increase the company’s operational efficiency to improves its margins, for a relatively low investment in tech.
HOW IT’S DONE
To make predictions about asset failures with AI we start by training a prediction model on the sequence of events that led to the failure by collecting the data corresponding to the failure. This data originates from sensors on the asset and is transported by the internet of things to the model, generally constructed in the cloud. Once our failure prediction model is trained by past failures, we can pattern-match the incoming data with the data represented by our AI model. If there is a close enough match between new data and the failure signature, a failure prediction is made with a certainty proportional to the closeness of the match.
Contact us if you’d like to understand more or click calendar to schedule a free 30-minute consultation if you have a company to improve.